Build the Most Powerful Data-Centric Applications with Kalray
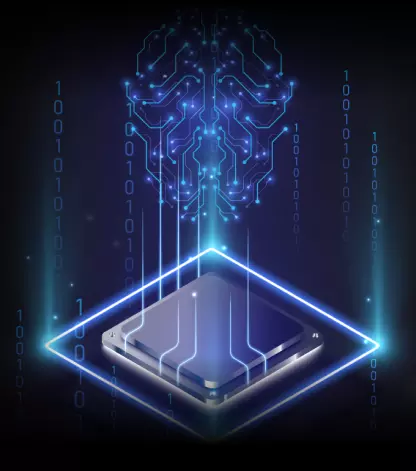
Home / Solutions
MPPA DPU Use Cases

Machine Learning
Why DPU for Machine Learning?
- Offload computational AI workloads
- Unprecedented programmability
- Best performance per watt and per $
Training & Inference
Machine learning enables computers to learn from data and make predictions or decisions without being explicitly programmed. The application of machine learning is a multi-step process: the first step, training the model with a reference labeled data set, adapts the parameters to the expected function. In the second step, called inference, the trained model is deployed and applied to new data, to enable decision making.
Offloading GPUs
From an implementation standpoint, training takes place offline, in data centers on servers accelerated by GPU-like processors or specialized hardware. For the inference part of AI, these processors are not sufficient, and here is where DPUs make the difference: Kalray DPUs enhance AI inference by efficiently offloading and accelerating specific tasks, such as image processing and data preprocessing, resulting in faster and more power-efficient AI model execution.
DPU Acceleration
Kalray’s acceleration cards provide the capability to efficiently and effectively offload the host CPU/GPU. Based on its MPPA® DPU processor, Kalray’s K200 family of data‐centric acceleration cards offer an unprecedented level of performance and programmability for AI inference workloads, a game changer solution in terms of performance per Watt and per dollar.
Smart Vision
Why DPU for Smart Vision?
- Offload computational AI workloads
- Unprecedented programmability
- Best performance per watt and per $
Capture, Process, Interpret
Smart vision is an AI application that utilizes computer vision techniques to enable machines to capture, process, and interpret visual information from the physical world, enabling tasks like image analysis, object recognition, and quality control. Smart vision applications are deployed across all industries.
Sustainable Manufacturing
With AI enabled smart vision, we are witnessing the biggest progression in automation since the introduction of the moving assembly line. Smart vision applications have a major impact on society as they enable smarter and safer cities with more efficient transport, they help us reduce our footprint with more sustainable manufacturing processes and they allow us to better respond to new diseases and provide better healthcare.
Boost Efficiency
Kalray DPUs play a crucial role in enhancing machine vision capabilities. By offloading computational workloads from the CPU or GPU, they significantly improve the speed and efficiency of smart vision applications. Kalray DPUs are particularly valuable in edge computing scenarios, where low latency and high throughput are essential, enabling devices like cameras and drones to process images and make intelligent decisions locally without relying heavily on cloud-based resources. This efficiency boost not only enhances the real-time capabilities of smart vision systems but also conserves energy and reduces the latency associated with cloud-based processing.
5G/Edge Computing
Why DPU for 5G/Edge Computing?
- Optimized for edge computing scenarios
- Analyze data where it is generated
- Best performance per watt and per $
Processing At the Edge
Edge computing is booming and thanks to the possibilities created by 5G networks, the growth of edge computing will accelerate exponentially. We talk about edge computing when data is processed and stored closer to the sources of data, rather than transferring the data to central data centers or the cloud. Organizations process data at the edge when latency is critical or to conserve bandwidth.
Maximize Efficiency
Data centers at the edge are typically limited in capacity and as such need maximal efficiency. Kalray enables customers to process data at the edge smarter, faster and more efficiently. Kalray’s patented MPPA DPUs and acceleration cards provide the capability to capture and perform inline analysis of a very large amount of data, close to where data is generated, to extract useful information from this flow of data and to react in real time based on this data.
Intelligent Data Centers
Why DPU for Intelligent Data Centers?
- Heterogenous multi-processing
- Offload host CPUs/GPUs
- Best performance per watt and per $
Performance & Efficiency
Data centers are growing massively due to the surge of data to be processed, applications to process the data and users who need to access these applications, often from remote locations. As a result, data center managers are under constant pressure to meet performance, scalability and efficiency requirements. To continue bringing high value to their demanding customers, data centers are seeking composable, highly efficient and heterogeneous, multi-processing and adaptive solutions, both on storage nodes and compute nodes.
Multiple Workloads
General purpose CPUs have hit their physical limitations as they can only support single threaded user applications. GPUs were not designed for dealing with multiple workloads and their data efficiently. Intelligent data centers need a new class of processing accelerator to efficiently run the predominantly data-centric heterogeneous processing tasks and offload main CPUs.
NGenea Use Cases
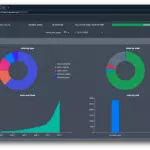
Generative AI
Why NG-Hub for Generative AI?
- Efficient support of data intensive AI workloads
- Global view of all unstructured transfers
- Best performance per watt and per $
Content Generation
Generative AI is an AI application that creates models capable of generating new content, be it in the form of text, graphics, music, or more. These models are typically powered by deep learning techniques like GANs (Generative Adversarial Networks) or RNNs (Recurrent Neural Networks). They can generate content that closely resembles human-created data. Generative AI significantly augments capabilities to produce novel, data-driven outputs and has found applications in a wide range of industries.
Large Data Sets
Generative AI applications require fast and seamless data access, especially when dealing with large data sets. The sheer volume of data required to train and fine-tune generative models can strain traditional storage systems, leading to performance bottlenecks and prolonged model training times. To make things even more challenging, data sets typically include a mix of large and small files and objects. Additionally, data format compatibility can be a hurdle, as generative AI models often require data in its native format for optimal performance. Lastly, managing and scaling storage infrastructures to accommodate the ever-growing data demands of generative AI applications can be complex and resource-intensive. Hybrid cloud offers the benefit of virtually unlimited scalability but requires fast data movement between on-premises and cloud infrastructures.
Automated Transfers
Kalray Ngenea addresses the storage challenges of Generative AI: NG-Hub, Ngenea’s data management layer, enables automated data transfer between on-premises and cloud storage and supports all data sizes. Intelligent caching minimizes data transfer costs and ensures instant data access. NG-Stor features a high-performance parallel file system that can easily manage petabytes of data, supporting the most demanding AI models. As such, Kalray Ngenea provides the best performance, scalability and efficiency for Generative AI data management.
Workload Acceleration
Why NG-Stor for Workload Acceleration?
- Powered by a high-performance parallel file system
- Manage petabytes of data and billions of files
- Highest throughput & IOPS/$ and lowest latency
High-performance Workloads
New workloads are entering the enterprise storage space: next-gen processors power innovative AI/ML, HPC, and Video applications. Enterprises need to maximize the processing cycles of their expensive compute infrastructure through low-latency, high-performance Tier-0 storage.
Parallel File System
Powered by a proven high-performance parallel file system trusted by thousands of organizations worldwide, NG-Stor can easily manage petabytes of data and billions of files, all under a single global namespace. NG-Stor meets the storage requirements of the most demanding HPC, AI/ML and post-production workloads. It leverages the fastest components (NVMe) to feed the most data-hungry compute processors and delivers the highest throughput & IOPS/$ and lowest latency.
Global Collaboration
Why NG-Stor for Global collaboration?
- Single Global Namespace
- Powerful search across all unstructured data
- Data is instantly available where needed
Access Any Data
Quickly and easily provide applications and users access to any data in the global environment, regardless of where they’re located.
Remote Teams
Cloud storage continues to be a big topic for enterprises: a key use case is global collaboration, to support distributed applications and remote teams. Global collaboration requires a single global namespace that allows remote users and applications to instantly access and share data across all storage tiers.
Single Namespace
Ngenea’s NG-Hub enables organizations to securely share data between remote teams, across sites. It creates a single namespace with supported (S3) CSPs and provides instant data access for distributed applications and remote users. Ngenea features real-time availability, powerful search and instant data access. It supports on-premises clouds as well as AWS, Azure and GCP.
Cloud Bursting
Why NG-Hub for Global collaboration?
- Single Global Namespace
- Powerful search across all unstructured data
- Data is instantly available where needed
Location Agnostic
Gain control to fully and easily leverage resources no matter where they’re located. Burst to the cloud when needed and scale back when done.
Reduces Costs/Instant Data Access?
Cloud bursting for data-intensive workloads requires organizations to quickly serve relevant data to compute instances in the Cloud. NGenea’s NG-Hub enables organizations to automatically transfer data from on-premises storage into the cloud, without manual data movement. There is no limit on the number of cloud compute instances and intelligent caching minimizes data transfer costs. NG-Hub provides each cloud compute instance with an easy access protocol, adheres to on-premises ACLs and security and only retrieves required data to reduce egress costs.
Scale as You Need
When on-premises infrastructures reach peak capacity, organizations “burst” extra workloads to public cloud services. This is a convenient and cost-effective method to support workloads with fluctuating demand patterns. Public cloud enables organizations to easily scale up or down to meet workload demands and are available worldwide. Cloud bursting enables organizations to reduce additional investment in on-premises infrastructure while leveraging the scale and flexibility of public cloud solutions. As such, they avoid service interruption to business-critical applications due to sudden workload spikes.
Scale-out NVMe Storage
Why NG-Box for Scale-out NVMe Storage?
- Designed for the most demanding workloads
- Easy to deploy and to use
- Best performance per Watt and $
NVMe Flash
The move from HDD to SSD created a whole new generation of much faster all-flash arrays. But traditional SSDs use SATA interfaces, which were designed for HDD and were not optimized for fast I/O. Today’s generation NVMe SSDs is much faster than traditional (SATA) SSD as the NVMe interface unlocks high IOPs, high bandwidth and ultra-low latency.
Disaggregation
Kalray’s NG-Box is a disaggregated NVMe storage array that was designed from the ground up to leverage the full potential of NVMe flash devices at massive scale, while ensuring the lowest storage Total Cost of Ownership (TCO). Featuring the Kalray K200-LP Smart Storage Controllers, Flashbox enables customers to deploy NVMe with the convenience of traditional storage array architectures, without performance or durability trade-offs.
Scaling NVMe
However, NVMe flash is more difficult to deploy at scale as traditional storage controllers cannot handle the NVMe performance and as such become performance bottlenecks. Some vendors designed scale-out NVMe solutions based on X86 but those do not have the convenience of traditional arrays, which customers have come to like a lot over the past decades.